Kernel Based Algorithms for Mining Huge Data Sets Supervised, Semi-supervised, and Unsupervised Learning
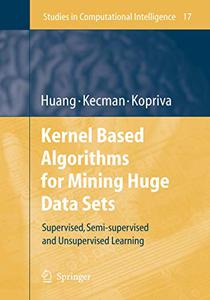
Kernel Based Algorithms for Mining Huge Data Sets: Supervised, Semi-supervised, and Unsupervised Learning by Te-Ming Huang , Vojislav Kecman , Ivica Kopriva
English | PDF(True) | 2006 | 266 Pages | ISBN : 3540316817 | 5.2 MB
"Kernel Based Algorithms for Mining Huge Data Sets" is the first book treating the fields of supervised, semi-supervised and unsupervised machine learning collectively. The book presents both the theory and the algorithms for mining huge data sets by using support vector machines (SVMs) in an iterative way. It demonstrates how kernel based SVMs can be used for dimensionality reduction (feature elimination) and shows the similarities and differences between the two most popular unsupervised techniques, the principal component analysis (PCA) and the independent component analysis (ICA). The book presents various examples, software, algorithmic solutions enabling the reader to develop their own codes for solving the problems.